News
Preferred Networks builds MN-2, a state-of-the-art supercomputer powered with NVIDIA GPUs.
2019.03.18
It will become operational in July to provide a combined computing power of 200*1 PetaFLOPS*2.
March 18, 2019, Tokyo Japan – Preferred Networks, Inc. (PFN, Head Office: Tokyo, President & CEO: Toru Nishikawa) will independently build a new private supercomputer called MN-2 and start operating it in July 2019.
MN-2 is a cutting-edge multi-node GPGPU*3 computing platform, using NVIDIA(R) V100 Tensor Core GPUs. This, combined with two other PFN private supercomputers ― MN-1 (in operation since September 2017) and MN-1b (in operation since July 2018), will provide PFN with total computing resources of about 200 PetaFLOPS. PFN also plans to start operating MN-3, a private supercomputer with PFN’s proprietary deep learning processor MN-Core(TM), in spring 2020.
By continuing to invest in computing resources, PFN will further accelerate practical applications of research and development in deep learning technologies and establish a competitive edge in the global development race.
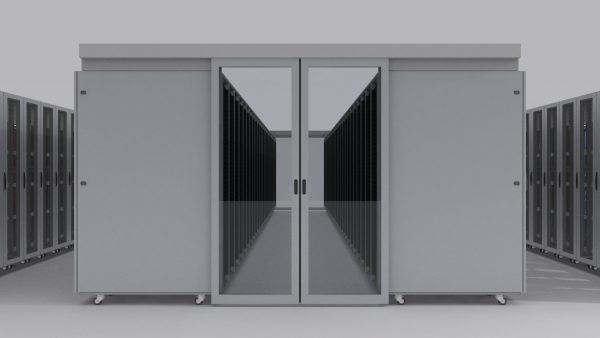
Conceptual image of the completed MN-2
Outline of PFN’s next-generation private supercomputer MN-2
MN-2 is PFN’s private supercomputer equipped with 5,760 latest CPU cores as well as 1,024 NVIDIA V100 Tensor Core GPUs and will be fully operational in July 2019. MN-2 is to be built on the premises of Yokohama Institute for Earth Sciences, Japan Agency for Marine-Earth Science and Technology. MN-2 will not only work with MN-3, which is scheduled to start operation in 2020 on the same site, but also connect with MN-1 and MN-1b, MN-2’s predecessors that are currently up and running, in a closed network. MN-2 can theoretically perform about 128 PetaFLOPS in mixed precision calculations, a method used in deep learning. This means that MN-2 alone has more than double the peak performance of MN-1b.
Each node on MN-2 has four 100-gigabit Ethernets, in conjunction with the adoption of RoCEv2*4, to interconnect with other GPU nodes. The uniquely tuned interconnect realizes high-speed, multi-node processing. Concurrently, PFN will self-build software-defined storage*5 with a total capacity of over 10PB and optimize data access in machine learning to speed up the training process.
PFN will fully utilize the open-source deep learning framework Chainer(TM) on MN-2 to further accelerate research and development in fields that require a large amount of computing resources such as personal robots, transportation systems, manufacturing, bio/healthcare, sports, and creative industries.
Comments from Takuya Akiba,
Corporate Officer, VP of Systems, Preferred Networks, Inc.
“We have been utilizing large-scale data centers with the state-of-the-art NVIDIA GPUs to do research and development on deep learning technology and its applications. High computational power is one of the major pillars of deep learning R&D. We are confident that the MN-2 with 1,024 NVIDIA V100s will further accelerate our R&D.”
Comments from Masataka Osaki,
Japan Country Manager, Vice President of Corporate Sales, NVIDIA
“NVIDIA is truly honored that Preferred Networks has chosen NVIDIA V100 for the MN-2, in addition to the currently operating MN-1 and MN-1b, also powered with our cutting-edge GPUs for data centers. We anticipate that the MN-2, accelerated by NVIDIA’s flagship product with high-speed GPU interconnect NVLink, will spur R&D of deep learning technologies and produce world-leading solutions.”
*1: The figure for MN-1 is the total PetaFLOPS in half precision. For MN-1b and MN-2, the figures are PetaFLOPS in mixed precisions. Mixed precisions are the combined use of more than one precision formats of floating-point operations.
*2: PetaFLOPS is a unit measuring computer performance. Peta is 1,000 trillion (10 to the power of 15) and FLOPS is used to count floating-point operations per second. Therefore, 1 PetaFLOPS means that a computer is capable of performing 1,000 trillion floating-point calculations per second.
*3: General-purpose computing on GPU
*4: RDMA over Converged Ethernet. RoCEv2 is one of the network protocols for direct memory access between remote nodes (RDMA) and a method to achieve low latency and high throughput on the Ethernet.
*5: Software-defined storage is a storage system in which software is used to centrally control distributed data storages and increase their utilization ratios.
*MN-Core(TM) and Chainer(TM) are the trademarks or registered trademarks of Preferred Networks, Inc. in Japan and elsewhere.